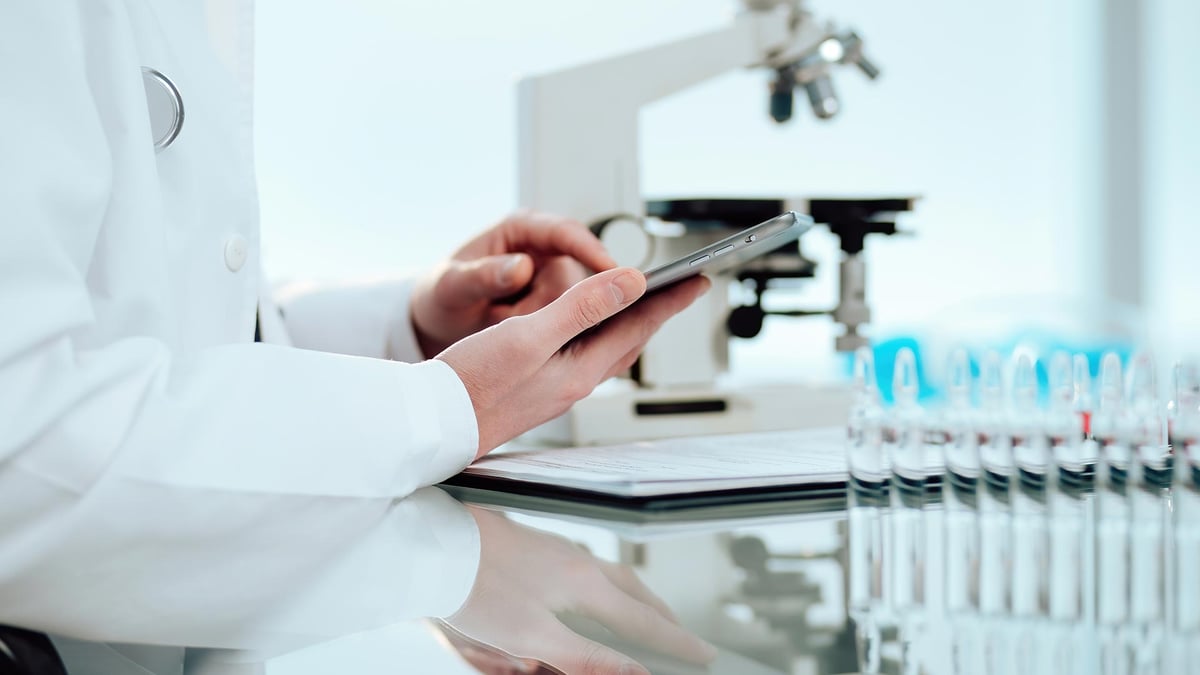
Data Quality… it's not a Goal, it’s a Mindset!
Despite investing huge amounts of money in setting up data collection and storage infrastructure, many companies are insight-starved, experience data leaks and are unable to formulate a Data Quality plan. Maybe the reason why is because Data Quality is seen as a goal rather than a mindset…
Defining the Often-Missed Aspects of Data Quality
Data Quality is often lost in translation when bigger ideas like data transformation and privacy are discussed. Data Quality only becomes part of the talk when an embarrassing mistake occurs, when insights and analytics give inaccurate results, when customers get angry, or when there’s a threat of a lawsuit because of data compliance violations.
What companies don't realize is that Data Quality is not some random objective to tick off. It’s a reality that is interwoven into the organization’s business process, its culture, its mindset, and its profitability. Businesses start with the goal of being data-driven, but they completely miss the process, the culture, and the intention along the way.
Ensuring Data Quality Standards
Data Quality is in worse shape than most managers realize. In a study conducted by the Harvard Business Review, only 3% of 75 executives found that their department fell within the acceptable range of correct data records. That's a staggering amount. So, what do companies do about this? Well, some of them use a basic data-cleaning process. Some employ expensive teams to clean the data. And others simply neglect bad data.
But high-quality data needs to be prioritized if a company wants to be successful. And there are ways to achieve this. First off, a company can conduct a data audit to evaluate the quality. Instead of detecting a failure, than hiring an expensive specialist to solve the issue, an organization can implement a Data Quality solution from the start. As an organization, it should establish a Data Governance Policy, set up a data-conscious culture, and provide data quality training. All this will help create a data quality mindset.
Reasons why life sciences should adopt a data-driven quality mindset
An organization with a genuine quality mindset goes over and above to ensure that quality is way beyond meeting compliance requirements. Adopting a data-driven quality mindset is one of the many initiatives that quality-oriented organizations follow.
In the past few years, there has been tremendous growth in developed technology based on data-driven models. Machine learning and artificial intelligence have revolutionalized everything around us. Data-driven models touch every aspect of our life, including the information we are exposed to on the internet. For example, based on our previous browsing history, we are exposed to several digital advertisements, such as movie suggestions, Instagram feeds, Twitter feeds, and Amazon suggestions.
So, can we also apply a data-driven quality mindset using similar algorithms? The answer is yes. Quality also has evolved from a hunch-based mindset to a data-driven, smart quality mindset. New technological developments like IoT and smart quality management systems are paving the way for data-driven decision-making in the life sciences. Data-driven quality mindset has several advantages over a traditional quality mindset.
Can't wait to know more about data-driven quality mindset and why organizations should apply it? Then scroll through the article, where we will discuss the top 5 reasons why life sciences should adopt a data-driven, quality mindset.
1. Proactive quality mindset
According to one statistic, the FDA recalls 1,279 drugs every year on average in the US. The number of recalled products is increasing at an alarmingly high rate and has invited criticism from the general public.
Product recalls can have disastrous consequences on patient health and the associated manufacturers, who incur huge losses. It also hurts the demand and supply dynamics, depriving the general public of availability of necessary medical supplies in the market. Therefore, the manufacturers must play a crucial role by building a proactive quality mindset, based on data-driven approaches.
By proactive, we mean that manufacturers need to change their mindset of analyzing the causes of failure after it has occurred. Instead there should be a quality mindset shift to stopping failure before it occurs. Predictive analytics can help manufacturers to achieve this goal.
2. Action-oriented quality mindset
Some life sciences companies deploy several sensors across their shop floor. The information coming from these sensors is very precious. The companies can use the sensor data to create predictive models based on machine learning principles. These data-driven models can predict future failure in advance, saving millions of dollars annually.
In addition to predicting future failure, data-driven predictive intelligence gives you actionable insights about what steps need to be taken to prevent failure. Due to the data-driven quality mindset, employees can have an on-the-spot action plan on what needs to be corrected to prevent failure. It also saves hundreds of hours that employees spend on retrospective root cause analysis and CAPAs.
Suppose your organization owns these highly sophisticated tools, such as NIR/FTIR sensors, high-end imaging tools, or even routinely deployed process sensors such as pH meters, temperature sensors, etc. In that case, it is high time to capitalize on them because a technology unused is as good as a technology that is unavailable.
3. Unbiased quality mindset
When we, as human beings, make any intuitive decision, our mind is biased - based on our emotions and previous experiences. All of us have different past experiences. Therefore, we tend to focus on our side of the story. On the contrary, data-driven decisions are purely based on logic. Additionally, when we make data-driven decisions, we are well aware of the statistic that quantifies the risk of making a wrong decision. Therefore, in a way, we are best prepared to face the possible consequences.
4. KPI-oriented quality mindset
The KPI-oriented quality mindset is slightly different from the previous approaches mentioned above because it focuses more on repeated failure / defect events instead of concentrating on isolated events of failures / defects.
It serves as an effective tool for top management to align the resources on products / processes where repeated failures / defects are occurring. For instance, if actionable insights show that the cause of repeated failures / defects is associated with the wear and tear of the manufacturing equipment, then management can make arrangements for necessary repairs or new purchase orders. Similarly, if the actionable insights show that the cause of failure / defect is associated with an untrained employee, then the management can make necessary training arrangements for the concerned employees.
The KPI-based quality mindset is also helpful for creating internal benchmarks for keeping employees motivated. For example, an organization can aim to meet the benchmark of 'zero batch recalls' for one of its best-selling products. And then, when employees overview the batch recall KPI in every periodic meeting, their progress on the target will keep them motivated to do better until they meet the benchmark.
In this manner, organizations can create and track different quality KPIs for different types of products, processes, defects, and failures.
The KPI-oriented quality mindset is important in organizations with massive product pipelines as, depending on the product value and frequency of occurrence of defects or failures, employees can focus their energies on a few vital projects rather than many trivial ones.
Another advantage of a KPI oriented quality mindset is that it can be incorporated as a factor in employee performance scorecards to keep the employees motivated to do better than best.
5. Competitive quality mindset
We live in a highly competitive world, and capability enhancement is one of the most efficient ways of beating the competition. According to a Mckinsey survey, AI adoption increased from 50% in 2020 to 56% in 2021. However, the business functions where AI is most common are service operations, product and service development, and marketing and sales. It means AI adoption for quality management is still in its infancy. And as we all know, in today's highly competitive world, the fastest to adopt is the first to win. Therefore, if you see a lot of your competitors still unaware of the opportunities presented by the data-driven quality mindset, then it is an opportunity for you to beat the competition by adopting the concept in your organization first.
Takeaways:
5 Points to Remember When Embracing a Data-Driven Mindset
Starting your journey towards a data-driven mindset comes with challenges but here are five takeaways so that you can overcome them. First, don't get lost when gathering data. Initially, companies tend to gather all their data in one huge lake or warehouse. Instead of centralizing all the data in one place, focus on the data that is essential for your business. Second, technology shouldn’t drive business. Business should drive technology. Machine learning and AI are exciting tools, but they should be guided by business goals, not the other way around.
Third takeaway. While data is good, data with context is even better. Remember, numbers are not enough on their own. Do they match expectations? What was the cause of meeting or not meeting those expectations? And what actions can be taken? Fourth. More is not necessarily better. We have more data available to us than ever before, but how much does your customer really need to see? Pinpoint the data that impacts them. And takeaway number five. Small victories lead to bigger success. Don’t wait for all the data to be centralized in one repository. See what initial value can be provided for each team within your organization.
Conclusion
Solving quality-related problems in the life sciences industry can take months or even years and is seen as an employee energy-draining activity. However, with a data-driven quality mindset, organizations can adopt a proactive approach to solving quality problems even before they occur. The data-driven mindset will win over the traditional quality mindset as it brings a lot of value to the table by saving time, money, and energy that goes into solving quality problems retrospectively.
In September 2021, the FDA published its plans for Data and IT modernization efforts with the new Office of Digital Transformation (ODT). The FDA states that this reorganization aims to protect and promote public health by improving its regulatory processes. The FDA looks forward to streamlining its operations and reducing duplicative processes through data and IT modernization. Needless to say, when the FDA itself resorts to data-driven decision-making, the life sciences manufacturers are expected to do the same.
Therefore, in the near future, we will see many organizations adopting a data-driven quality mindset where almost all employees will leverage data to make quality-related decisions. As a result, organizations will see fewer product recalls, and employees will be free to focus more on innovation and new product development.
Discover how a Smart Quality Platform can help you instill a data-driven quality mindset!