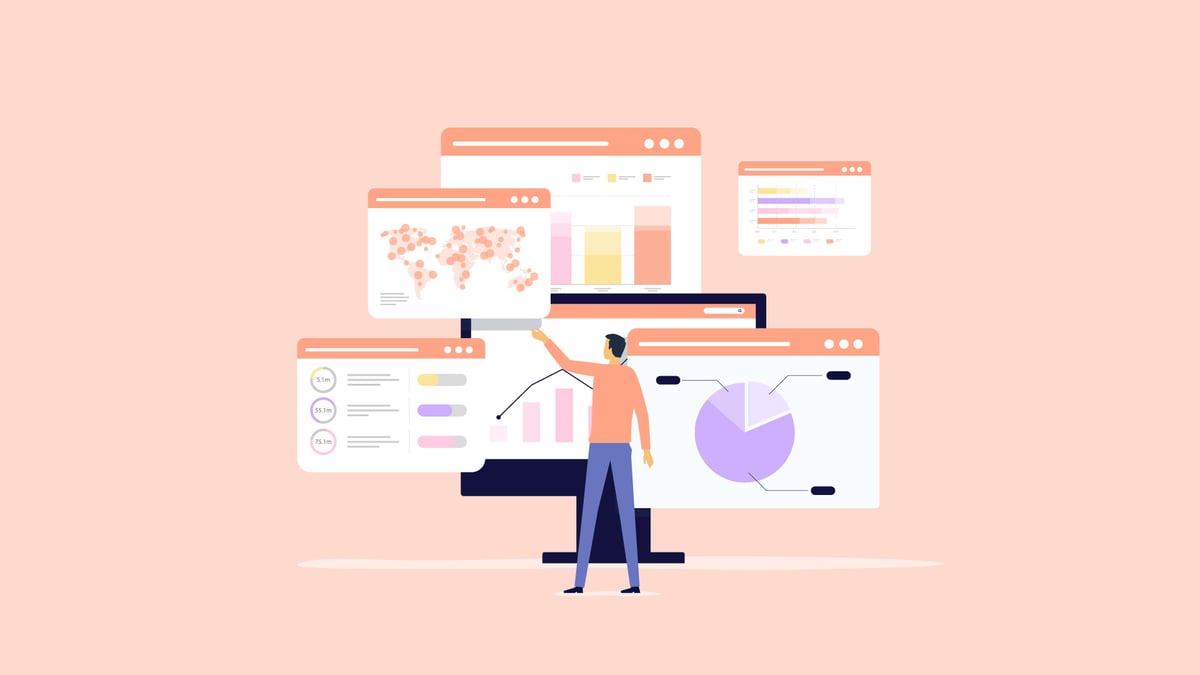
As the life sciences are digitalizing at an unprecedented scale and rate, data lifecycle management is becoming increasingly important. The two major factors driving this rapid and close-to-universal digitalization are Industry 4.0 and the COVID-19 pandemic. While not unique to our industry, these two elements are greatly impacting the life sciences in unparalleled ways.
Industry 4.0 encourages automation through intelligent systems based on data-driven software technologies. Therefore, many companies in the life sciences are actively working on digitalizing scientific data. At the same time, the recent pandemic has caused many organizations to switch to a hybrid work mode, and many retained this new mode of working after the spread of the disease was controlled. Of course, most life sciences companies are in the manufacturing business, making it impossible to function in 100% remote mode. Still, communication across teams can improve if your employees are enabled with digital tools such as an e-Quality Management System, e-Lab Notebook, and e-Batch Manufacturing Records.
Today, almost team members in a life sciences company handle data in varying capacities. Considering the importance of data integrity regulations in the industry, it is crucially important to implement best practices for proper data lifecycle management. This article discusses eight stages of data lifecycle management to help you get started with the best practices in your company.
Stage 1: Generation
The first step to managing your data lifecycle successfully is data generation. In the life sciences industry, data generation is an ongoing process resulting from R&D, compliance, and manufacturing records. Some of the important activities that lead to the generation of the data are:
- Raw material specifications
- Raw material inventory
- R&D experiments and manufacturing batch records
- In-process and finished product test results for R&D experiments
- Compliance data of R&D activities
- Batch release data
- Marketing and sales data
- Finance data
Stage 2: Collection
The data generation activities in the first stage of data lifecycle management lead directly to data collection. This may sound simple, but collecting large chunks of data accurately is quite the challenge! This is where software-driven automation can come into play. A systematically planned data policy may help you manage this step effortlessly. Some of the leading software solutions to collect data are listed below:
Function |
Data Type |
Software Solution Examples |
Retail Stores |
|
SAP ERP, Odoo Enterprise |
R&D |
|
MasterControl e-Lab Notebooks |
Manufacturing |
|
e-Batch Manufacturing Records |
Compliance |
|
Scilife e-Quality Management System |
Stage 3: Storage
Now that your data is available in an accessible and usable form, the next step in the data lifecycle management is to ensure that it is stored safely for future use. Depending on your organization's IT policies, you can store your data using off-site cloud services such as Data Historian Platform, or on-site options like SSDs, hard disk drives, magnetic LTO tape, or even DVDs. One very important factor for on-site storage methods is to consider their conservatively expected lifespan.
Stage 4: Processing
Once data has been collected and digitalized with the help of your preferred software services, it must be processed. A good data lakehouse can help you achieve this step. Data processing may include – but is not limited to – the following activities:
-
Data wrangling
in which data from various software sources is combined in a meaningful way to clean and transform data from raw form into a more accessible and usable format. -
Data compression
is an essential processing step in which data is transformed to be more efficiently stored. -
Data encryption
is another data processing step in which data is translated into another form of code to protect it due to privacy concerns. -
Data processing
may also include the simple act of converting printed data into digital data.
Stage 5: Management
Just storing data is not enough! That’s why this next step in the data lifecycle is to manage all stored data. Backing up your data is one important step to protect data from unintentional damage or loss. Your data backup strategy should address the following questions:
- Who will be responsible for managing data backups?
- Will it be an automated process?
- How frequently will the backup take place?
- How many copies of the backup will be created?
- Where will the backup be stored?
- Who will have access to the backup?
Another important aspect of data management is defining user access levels. This may include particular info about data viewing, editing, analyzing, and deleting rights of users based on their authority level.
Stage 6: Analysis
This step is truly the core of the data lifecycle. Here, data analysts perform various tests on the data to derive meaningful insights. Your analysts may perform several graphing options on the data, followed by data modeling using machine learning, artificial intelligence, statistical methods, or mathematical methods. The data analysts will also select the most appropriate data analysis technique based on the problem statement they are trying to solve. This may include performing model validation tests to finalize the model for future predictions based on your data.
Stage 7: Visualization & Interpretation
Once you have successfully validated the model in step 6, you can move on to Data Visualization. The purpose of this step is to represent the information contained in the data in a palpable format. Your data analyst may create various data visualization dashboards to help users visualize the data. For example, one data visualization dashboard can highlight manufacturing process differences in the out-of-specification batch vs. the successfully released batch.
Users derive meaningful insights from the charts and predictions displayed on data visualization dashboards. This data interpretation is used for important business decisions and process improvements.
Stage 8: Destruction
It is crucial that data destruction (also known as data purging) also removes every obsolete copy of an item. This is typically done in an archive storage location. The challenge of this phase of the lifecycle is to ensure that all data has been properly destroyed. But even before destroying data, it is important to ensure that the targeted items have exceeded their regulatory minimum retention period.
In the Life Sciences, companies must retain their data records according to all applicable regulations for the specific products they manufacture. For example, products manufactured in or exported to the USA need to comply with general record-keeping requirements described in § 211.180, meaning records must be retained for at least one year after the expiration date of a batch and for certain over-the-counter (OTC) drugs for at least three years after distribution of the batch (§ 211.180(a)).
Once this period ends and data is no longer required to be retained, that data no longer serves a meaningful purpose to your organization. Therefore, it can be safely removed from storage archives. However, before removing the data, manufacturers should also ensure that – apart from the mandatory record retention period – the targeted data is not being actively used as a benchmark (internal standard) or as calibration data for a machine learning model deployed in real-time quality control procedures for assessing or predicting future outcomes.
Conclusion
Your systematic approach to data lifecycle management is instrumental in driving data-based decisions for your organization. Interlinking and automating these eight steps for an effective and efficient data lifecycle management will help you stay focused on the ultimate goal of data interpretation. Data truly is the ‘new oil’ of the 21st Century – and it is up to you to make it the driving force of your organization’s future.